Single Point¶
janus-core
contains various machine learnt interatomic potentials (MLIPs), including MACE based models (MACE-MP, MACE-OFF), CHGNet, SevenNet and more, full list on https://github.com/stfc/janus-core.
Other will be added as their utility is proven beyond a specific material.
Set up environment (optional)¶
These steps are required for Google Colab, but may work on other systems too:
[1]:
# import locale
# locale.getpreferredencoding = lambda: "UTF-8"
# ! pip uninstall torch torchaudio torchvision transformers numpy -y
# ! uv pip install janus-core[all] data-tutorials torch==2.5.1 --system
# get_ipython().kernel.do_shutdown(restart=True)
Use data_tutorials
to get the data required for this tutorial:
[2]:
from data_tutorials.data import get_data
get_data(
url="https://raw.githubusercontent.com/stfc/janus-tutorials/main/data/",
filename=["sucrose.xyz", "NaCl-set.xyz"],
folder="data",
)
try to download sucrose.xyz from https://raw.githubusercontent.com/stfc/janus-tutorials/main/data/ and save it in data/sucrose.xyz
saved in data/sucrose.xyz
try to download NaCl-set.xyz from https://raw.githubusercontent.com/stfc/janus-tutorials/main/data/ and save it in data/NaCl-set.xyz
saved in data/NaCl-set.xyz
Single point calculations for periodic system¶
[3]:
from ase.build import bulk
from janus_core.calculations.single_point import SinglePoint
# Change to cuda if you have a gpu or mps for apple silicon
device = "cpu"
NaCl = bulk("NaCl", "rocksalt", a=5.63, cubic=True)
sp = SinglePoint(
struct=NaCl,
arch="mace_mp",
device=device,
model="small",
calc_kwargs={"default_dtype": "float64"},
)
res_mace = sp.run()
NaCl = bulk("NaCl", "rocksalt", a=5.63, cubic=True)
sp = SinglePoint(
struct=NaCl,
arch="sevennet",
device=device,
)
res_sevennet = sp.run()
NaCl = bulk("NaCl", "rocksalt", a=5.63, cubic=True)
sp = SinglePoint(
struct=NaCl,
arch="chgnet",
device=device,
)
res_chgnet = sp.run()
print(f" MACE[eV]: {res_mace['energy']}")
print(f"SevenNet[eV]: {res_sevennet['energy']}")
print(f" CHGNeT[eV]: {res_chgnet['energy']}")
/home/runner/work/janus-core/janus-core/.venv/lib/python3.12/site-packages/e3nn/o3/_wigner.py:10: UserWarning: Environment variable TORCH_FORCE_NO_WEIGHTS_ONLY_LOAD detected, since the`weights_only` argument was not explicitly passed to `torch.load`, forcing weights_only=False.
_Jd, _W3j_flat, _W3j_indices = torch.load(os.path.join(os.path.dirname(__file__), 'constants.pt'))
cuequivariance or cuequivariance_torch is not available. Cuequivariance acceleration will be disabled.
Using Materials Project MACE for MACECalculator with /home/runner/.cache/mace/20231210mace128L0_energy_epoch249model
Using float64 for MACECalculator, which is slower but more accurate. Recommended for geometry optimization.
Using head Default out of ['Default']
/home/runner/work/janus-core/janus-core/.venv/lib/python3.12/site-packages/mace/calculators/mace.py:143: UserWarning: Environment variable TORCH_FORCE_NO_WEIGHTS_ONLY_LOAD detected, since the`weights_only` argument was not explicitly passed to `torch.load`, forcing weights_only=False.
torch.load(f=model_path, map_location=device)
CHGNet v0.3.0 initialized with 412,525 parameters
CHGNet will run on cpu
MACE[eV]: -27.0300815358917
SevenNet[eV]: -27.057369232177734
CHGNeT[eV]: -29.32662010192871
Simple Molecules¶
[4]:
from ase.build import molecule
from weas_widget import WeasWidget
from janus_core.calculations.single_point import SinglePoint
sp = SinglePoint(
struct=molecule("H2O"),
arch="mace_off",
device=device,
model="medium",
)
res = sp.run()
print(res)
v=WeasWidget()
v.from_ase(sp.struct)
v.avr.model_style = 1
v.avr.show_hydrogen_bonds = True
v
Downloading MACE model from 'https://github.com/ACEsuit/mace-off/raw/main/mace_off23/MACE-OFF23_medium.model?raw=true'
The model is distributed under the Academic Software License (ASL) license, see https://github.com/gabor1/ASL
To use the model you accept the terms of the license.
ASL is based on the Gnu Public License, but does not permit commercial use
Cached MACE model to /home/runner/.cache/mace/MACE-OFF23_medium.model
Using MACE-OFF23 MODEL for MACECalculator with /home/runner/.cache/mace/MACE-OFF23_medium.model
Using float64 for MACECalculator, which is slower but more accurate. Recommended for geometry optimization.
Using head Default out of ['Default']
/home/runner/work/janus-core/janus-core/.venv/lib/python3.12/site-packages/mace/calculators/mace.py:143: UserWarning: Environment variable TORCH_FORCE_NO_WEIGHTS_ONLY_LOAD detected, since the`weights_only` argument was not explicitly passed to `torch.load`, forcing weights_only=False.
torch.load(f=model_path, map_location=device)
{'energy': -2081.11639716893, 'forces': array([[ 0.00000000e+00, -3.88578059e-16, -6.47486826e-01],
[ 0.00000000e+00, -3.43765601e-01, 3.23743413e-01],
[ 0.00000000e+00, 3.43765601e-01, 3.23743413e-01]]), 'stress': array([0.00000000e+00, 6.12631596e-06, 4.50763482e-06, 2.43028903e-21,
0.00000000e+00, 0.00000000e+00])}
[4]:
Sugar on salt¶
[5]:
from ase.build import add_adsorbate, bulk
from ase.io import read, write
a = 5.63
NaCl = bulk(
"NaCl", crystalstructure="rocksalt", cubic=True, orthorhombic=True, a=5.63
) * (6, 6, 3)
NaCl.center(vacuum=20.0, axis=2)
sugar = read("data/sucrose.xyz")
add_adsorbate(slab=NaCl, adsorbate=sugar, height=4.5, position=(10, 10))
write("slab.xyz", NaCl)
sp = SinglePoint(
struct="slab.xyz",
arch="mace_mp",
device=device,
model="small",
)
res = sp.run()
print(res)
v = WeasWidget()
v.avr.model_style = 1
v.avr.show_hydrogen_bonds = True
v.from_ase(NaCl)
v
Using Materials Project MACE for MACECalculator with /home/runner/.cache/mace/20231210mace128L0_energy_epoch249model
Using float64 for MACECalculator, which is slower but more accurate. Recommended for geometry optimization.
Using head Default out of ['Default']
/home/runner/work/janus-core/janus-core/.venv/lib/python3.12/site-packages/ase/io/extxyz.py:318: UserWarning: Skipping unhashable information adsorbate_info
warnings.warn('Skipping unhashable information '
/home/runner/work/janus-core/janus-core/.venv/lib/python3.12/site-packages/mace/calculators/mace.py:143: UserWarning: Environment variable TORCH_FORCE_NO_WEIGHTS_ONLY_LOAD detected, since the`weights_only` argument was not explicitly passed to `torch.load`, forcing weights_only=False.
torch.load(f=model_path, map_location=device)
{'energy': -1706.3958128381037, 'forces': array([[ 1.52358986e-09, 1.52359060e-09, 1.60311833e-01],
[ 5.89516217e-10, 5.89516399e-10, -2.49849858e-01],
[-3.71422142e-08, -4.76124738e-09, 2.55549362e-03],
...,
[-1.81414029e-01, 1.43043590e-01, -7.13535637e-02],
[ 1.37346116e-01, -1.33239451e-01, -1.05307659e-01],
[ 5.18585386e-02, 1.62407401e-01, -8.67263312e-02]]), 'stress': array([-4.33386986e-05, 7.45245471e-07, -1.69529958e-03, -5.32726537e-05,
-3.41297966e-05, -4.53043161e-05])}
[5]:
Calculate an entire collection of data frames¶
[6]:
from ase.io import read
import matplotlib.pyplot as plt
import numpy as np
frames = read("data/NaCl-set.xyz", format="extxyz", index=":")
dft_energy = np.array([s.info["dft_energy"] / len(s) for s in frames])
sp = SinglePoint(
struct="data/NaCl-set.xyz",
arch="mace_mp",
device=device,
model="medium-0b3",
)
sp.run()
mace_mp_energy = np.array([s.info["mace_mp_energy"] / len(s) for s in sp.struct])
rmse_mace = np.linalg.norm(mace_mp_energy - dft_energy) / np.sqrt(len(dft_energy))
sp = SinglePoint(struct="data/NaCl-set.xyz", arch="chgnet", device=device)
sp.run()
chgnet_energy = np.array([s.info["chgnet_energy"] / len(s) for s in sp.struct])
rmse_chgnet = np.linalg.norm(chgnet_energy - dft_energy) / np.sqrt(len(dft_energy))
sp = SinglePoint(struct="data/NaCl-set.xyz", arch="sevennet", device=device)
sp.run()
sevennet_energy = np.array([s.info["sevennet_energy"] / len(s) for s in sp.struct])
rmse_sevennet = np.linalg.norm(sevennet_energy - dft_energy) / np.sqrt(len(dft_energy))
print(
f"rmse: mace_mp = {rmse_mace}, chgnet = {rmse_chgnet}, "
f"sevennet = {rmse_sevennet} eV/atom"
)
fig, ax = plt.subplots()
ax.scatter(dft_energy, mace_mp_energy, marker="o", label="mace-mp-0")
ax.scatter(dft_energy, sevennet_energy, marker="x", label="sevennet")
ax.scatter(dft_energy, chgnet_energy, marker="+", label="chgnet")
ax.legend()
plt.xlabel("MLIP [eV/atom]")
plt.ylabel("DFT [eV/atom]")
plt.show()
Using Materials Project MACE for MACECalculator with /home/runner/.cache/mace/macemp0b3mediummodel
Using float64 for MACECalculator, which is slower but more accurate. Recommended for geometry optimization.
Using head default out of ['default']
/home/runner/work/janus-core/janus-core/.venv/lib/python3.12/site-packages/mace/calculators/mace.py:143: UserWarning: Environment variable TORCH_FORCE_NO_WEIGHTS_ONLY_LOAD detected, since the`weights_only` argument was not explicitly passed to `torch.load`, forcing weights_only=False.
torch.load(f=model_path, map_location=device)
CHGNet v0.3.0 initialized with 412,525 parameters
CHGNet will run on cpu
rmse: mace_mp = 0.06372546945345034, chgnet = 0.41196170963560735, sevennet = 0.02978418682071067 eV/atom
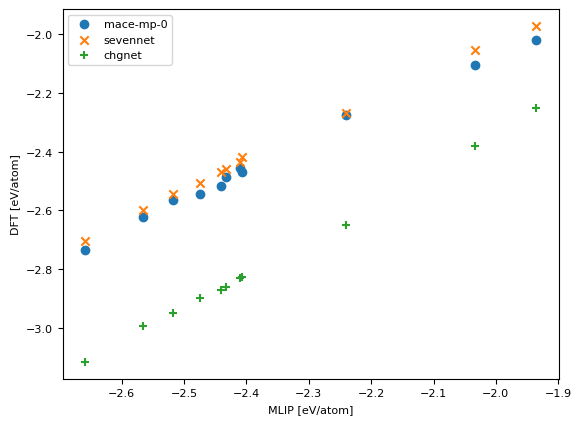